Shyam Gollakota, a professor at the University of Washington and digital health innovator, is pioneering a revolutionary solution to the ‘cocktail party problem,’ a longstanding challenge in hearing technology. His team has created a groundbreaking “sound bubble” device that enables users to amplify voices within a specific range while minimizing external noise and distractions. Designed to adapt dynamically to different listening environments, this technology provides a customizable solution for clearer communication in noisy settings.
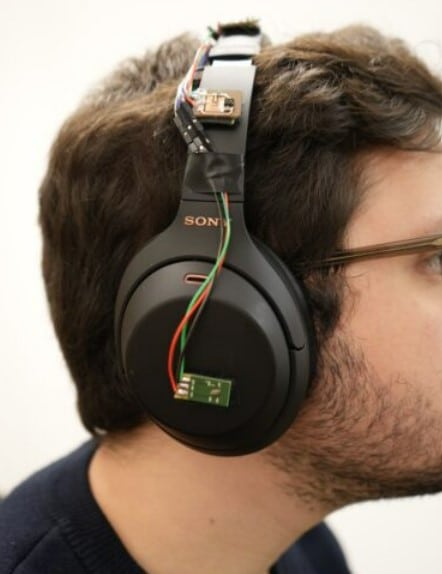
The team developed a prototype using standard headphones equipped with microphones, as shown in this image. (Credit: Chen et al./Nature Electronics)
The device allows users to focus on voices within the bubble, filtering out unwanted sounds for an improved auditory experience. By isolating and enhancing targeted speech, the sound bubble provides a transformative way to navigate challenging auditory environments.
The current prototype, a headset equipped with six microphones, leverages advanced AI algorithms to achieve precise sound localization and selective voice amplification. This system dynamically adjusts in real time, enabling users to modify the bubble’s size and shape without requiring individual calibration. The technology’s versatility makes it suitable for a wide range of applications, from improving everyday conversations to providing specialized support for individuals with hearing impairments. Gollakota also envisions integrating the technology into wireless earbuds and hearing aids, ensuring accessibility and convenience for broader use cases.
Looking ahead, Gollakota’s team is focused on scaling this innovation for both consumer and healthcare markets. While portions of the algorithm are open-sourced to encourage further research and collaboration, commercialization efforts aim to bridge hardware and software expertise to accelerate adoption. Beyond hearing enhancement, Gollakota envisions hearables evolving into a natural interface between humans and AI, with applications in augmenting memory, creativity, and intelligence. His work underscores the potential of wearable audio technology to transform not only how we hear but also how we interact with the world around us.
Full Episode Transcript
Hello, everyone, and welcome to This Week in Hearing. A few podcasts ago, on the topic of AI speech and noise separation, I said that I don’t need to tell anyone that solving the cocktail party problem is one of the biggest challenges for hearing device makers. The one after that I said I no longer remember how many times I’ve opened a podcast by pointing out that speech in noise is the last frontier in hearing device performance. The podcast after that, I just gave up and we got right into the details. The interesting thing is, despite all the activity, there’s still room for different approaches. For example, straight speech in noise separation doesn’t work if there’s a loud person nearby. That’s making it hard to hear your companion. That’s where today’s guest comes in. Shyam Gollakota is a professor at the Paul G. Allen School of Computer Science and Engineering at the University of Washington and a serial entrepreneur in digital health. Shyam, before we get into your team’s approach to the cocktail party problem, please tell everyone a bit about your history and background. Yeah. Thank you so much Andrew, for having me on this podcast. I’m a professor at the University of Washington in the computer science department and have been pretty interested in how we can use mobile technologies and more recently AI to really create new human AI interfaces and human AI augmentation of our senses. And in the case of what we’re going to talk about today, how do you create something like super hearing or enhancing our hearing capabilities? This can be pretty transformative because that’s where AI and humans can be augmented to enhance the way we sense our environment. And that’s a pretty exciting future in my opinion. Oh, I quite agree. And as an actual hearing impaired person, these improvements in use of AI for the hearing case haven’t come fast enough and I’m sure a lot of people will agree. Now, in particular, you’ve prototyped a device which creates a sound bubble which allows voices inside the bubble to be heard while rejecting voices outside of it. Is that correct? That is correct. So I think the whole idea behind this came when we went to restaurants to have a group meeting. These days restaurants are super noisy. They’re also packed. So you know that, like, it’s really hard to focus on the. I’m always asking the person at my table, what did you just say? Again, I can’t really hear you properly. And as I’m getting older, it becomes harder to hear as well. So imagine you’re being in such a busy Restaurant and having the ability to listen to everyone at your table, but suppress all the speakers and the noise who are not close to the table. That’s exactly what we did here. We created a headphone device that allows the listeners to create what we are calling a sound bubble around them where speakers outside the bubble are kind of suppressed and noise outside the bubble is suppressed. But this bubble can be said to be three to five feet. But then people inside the bubble or sounds from inside the bubble can be heard very clearly. Okay, so you can adjust the size of the bubble going from 3ft, roughly a meter, to say 6ft, 2 meters and anything in between. Yeah, so we started off by saying, okay, so let’s start with the fixed bubble size, which is, let’s say we set it to 1.5 meters, for example. And then we were like, okay, maybe we can actually do multiple bubble sizes. So we did three different discrete bubble sizes, 1 meter, 1.5 meter and 2 meters. And then we were like, do people really know what 1 meter means? People really know what 2 meters really means. So then we finally basically decided in the paper, we actually showed all these different things where we showed that we can actually set it to any arbitrary value between one and two meters. And so you can potentially have a slider where you can just increase a slider as long and you can increase the slider until the person who you want to hear is within the said bubble without having to figure out what is 1 meter, what is 1.5 meters, what is 2 meters, and so on. Okay, nice. And I should mention that we’re going to put in the show notes two things. One is your peer reviewed paper in Nature. And then there was also a summary written and published by the university. We’ll include them both. Now when you talk about being able to adjust the bubble, the size of the bubble, how sharp is the border of the bubble, in other words, how quickly does sound fall off as someone migrates out of the bubble. So we did a pretty extensive study both in simulations and in the real world as well. And it turns out that this really depends on the room you are in and the reverberations of the room you are actually in. And that’s the reason why it’s really hard to say what exactly one meter is. But what we saw was that the variations across the rooms was around 10 cm to 15 to 20 cm. That was a range. So when we asked people to basically walk into the bubble and say when exactly you could suddenly start hearing the person in the bubble and not hear the person within the bubble. We saw that if the bubble was say 1.5 meters, that particular range was between 1.55 to 1.44 meters. So it was basically around 10 centimeters of variability. It could increase. If it’s a really reverberant room, it can go up to 20cm. But I don’t think that really a practical, practical application, whether it’s 10cm or 20cm, it is not really going to make a big impact because you’re just going to set it to like to include the person who is within your size and it’s just going to include all the sounds in that particular bubble. So the variability, the range is actually pretty good. But if somebody is just outside the bubble, how much has their voice fallen off? In other words, they have somebody who’s near the bubble but not in it. Is it a gradual decline or there’s a very sharp cliff where they’re either in the bubble or out? It is a pretty sharp decline in the sense that as soon as it’s interesting because right when you’re at the bubble things start becoming, you can start hearing the voice slightly and it’s like hearing some garbled voice of the person. But as soon as you enter, it almost feels like you’re entering a barrier to experience the whole thing. If you come here and wear the headphone itself and suddenly you hear the voice really clearly. But there is this there’s this interval of between 10-15 cm. It’s hard to really tell exactly what that duration is that distances. But during that distance you’re getting in and out of the person, you’re getting the voice in and out kind of. That’s basically the experience you’re seeing in practice where you’re getting a bit of the person and it’s kind of garbled. But as soon as you enter the bubble everything is perfectly clear. And as soon as you get out of that tiny region of 10 to 15 centimeters, everything is basically falls off completely. And I saw that you’re achieving 49 decibels of reduction outside the bubble versus within, which is a huge number. How much variability is there in that with regard to different rooms and so on. So that number is pretty consistent. This is based, so there are two different numbers. One of them is what the algorithm can achieve and what the person can here because you’re using a noise canceling headset. So the algorithm itself is very sharp in terms of just removing all the sounds which are outside the bubble. And it’s very consistent across the rooms that it’s basically about the 40dB threshold. You might have a variability above 40dB, but 40dB is almost pretty high. But the other limiting factor which people should be aware of is when you’re wearing noise canceling headsets, your algorithm might be canceling out everything and then playing it inside. But it’s also the fact that noise is leaking through your actually it’s not a perfect suction cup. So you’re still having some noise, sound leakage. So you’re kind of limited at that point by not the 40dB cancellation of the algorithm, but how good your noise canceling headphone really is. And that’s around 20 to 25 decibels. That’s basically what typical noise canceling headsets do. The story is of course different. You have, if you have hearing loss where you don’t need a noise canceling headset at that point. But yeah, that’s the touch of picture of what we’re able to achieve. The machine learning algorithm itself can basically eliminate the signal and people who are outside the bubble. It’s 40 to 50 decibels, but, and what’s being played into the ear removes all the sounds. But because the headsets are not perfectly sound isolating, you still have some leakage. And that’s basically the net effect is that you are getting around 20 to 25 decibels cancellation because of noise cancellation as well. Right. Okay. So yeah, you can see then how that affects a couple of different use cases. If I am a normal or say mildly hearing impaired person who has trouble hearing speech and noise I might use an enabled consumer earbud. And now you can get pretty good noise cancellation that will go deeper than 25 decibels and almost more important extend out into higher frequencies. So you’re canceling more of the voice spectrum and then your device is going to let in what I want within the bubble. Now if I’m more severely hearing impaired, well, and I am more severely hearing impaired, then you -really, what you’re doing is, is you’re providing some SNR improvement by only amplifying the voices within the bubble. I’m still going to hear more of everything, but the voices within the bubble will be amplified and the voices outside the bubble just be natural, pass through. So you’ll still get some speech and noise improvement. And I suppose at this early stage you haven’t studied how much that is. We are working on that right now. We are actually working on right now with like hearing loss patients as well to see how much improvement you’re getting. Of course, as you mentioned, it really depends on the degree of hearing loss and you might need different noise isolation based on the hearing loss as well. So we are considering, we are basically evaluating all those factors. But I think it goes back to one of the questions you basically raised, the way you introduced the whole thing saying that existing, almost everything out there is doing speech enhancement or noise reduction. All the, like the latest headphones or like the hearing aids, when they say they are AI, they are effectively all they are doing is basically reducing the amount of noise in the environment. The de-noising, this is what’s called denoising and basically amplifying the, the speech in the environment itself. But what is actually the harder problem is what, as you mentioned, the cocktail party problem, where you have a large number of different people, speakers who might be louder, who might be the similar amplitude and you’re trying to listen to a subset of speakers among these larger number of speakers in your environment. In the first place, this is not just a denoising, because denoising would just remove the noise, but let all the speakers in. But that’s not what you’re trying to do in, for example, in a restaurant scenario or in a cocktail party. So to say you’re listening to a specific set of people. And the question, it becomes a user interface problem, which is how does a user determine and tell the device which subset of users that the AI should actually focus on? Right. And a bubble is a very natural way to basically figure out which subset of speakers to focus on. Because typically when we have conversations, we are focused on people who are closer to us. Like at a restaurant, we are on a table or at a bar, we are talking to people who are next to us. So a bubble is a very natural user interface, in my opinion, to pick the subset of users or subset of speakers in the room who you want to actually listen to. And that’s the reason why this bubble is very exciting in terms of being a very simple. Simplistic user interface, but also going after and addressing this cocktail party problem, which goes beyond just denoising, which is what the products today on the market are basically trying to solve. Now that makes perfect sense. But it also raises a question. You use the. I was going to say for anybody who’s been in a cafe in Paris, you know how the tables are on top of each other. Your example in the bar, if I’m sitting at a bar stool facing my companion, and on the next bar stool over the same distance away, but behind me is a very loud person talking. So I’ll have to let them both in the bubble. Unless you can put some directionality to the bubble. Can you shape the bubble? For example, could I do a cardioid pattern which lets in things from the side to the front but not the back? Can you do that? That’s a really good question. And yes, we can do that. The reason why in this work we focused on the bubble is because when we started out this project, it was unclear if we can get distance perception. Primarily because humans, it’s well known that human auditory system is not really good at perceiving distance through sound. And so it is unclear if you can, primarily because of separation and everything else, you can get the directionality really well. You can actually directionality ability to figure out which direction the sound is coming from. People can do that really well. But if you basically have someone to close the eyes and figure out what distance the person is speaking at, it’s going to be really challenging, in particular for unknown environments and unknown speakers, primarily because people who are farther can be speaking louder than people who are actually closer. So you can’t just use amplitude information itself. So it was unclear when we started if we can actually get this distance perception in real time on an AI using an AI algorithm. And what we’ve shown that in fact you can actually learn the distance information not just for a single source, but multiple sources, all in real time within like less than 10 milliseconds and create this bubble. But once we have this distance, the bubble is basically another way of saying that we now have distance perception. You can now combine that with directionality. You can just say, have distance. I basically also want to only focus on in the forward direction. So I can basically create like shapes of all kinds which are not only just based on direction, directional, anger, but also based on the distance. So it doesn’t really just pick up. Just going back to your example, it’s not just also picking up someone who’s behind the person who I’m actually talking to is basically be limited to the person who I’m actually talking to and not in the backward direction, for example. So what we are showing here is that we can get distance perception. But now if you combine that with directionality you have a much finer grained control of creating zones of silence or zones of conversations which you can potentially program. Right, okay, so that’s really encouraging that you have created the algorithm that can actually separate or change the shape of the bubble and you can almost then reject voices at will through that, which is really interesting. Now, multiple questions come to mind. The first one is, what if you have two people speaking within the bubble or three people speaking within the bubble? Yes, we had to basically one of the good things about a bubble is basically just that like you conversations are not limited to a single person. And second, people are not always looking at. I’m sitting at a bar next to someone, I’m not looking at their face all the time. So directionality by itself will not really work. So you want to be able to be automatically in a situation where, you know all the sound sources, not just one, but all the sound sources across like a wider angle around you and let make sure that all of them are basically being heard. And that’s basically what we demonstrated in this work, which is that you can have three, up to three people inside the bubble and more than three to three people outside the bubble talking with equal amplitude, equal loudness. Sometimes you can actually have the people outside talking even louder. And we are still able to identify who is within the bubble and like play both the speakers even though they have overlapped. Let’s say when I’m speaking, you might be saying, I agree, that can, that can have an overlap. So it’s actually keeping both my speech as well as the overlap speech, as long as both of the people are within the bubble itself. Okay. All right. And the other question I had which along similar lines is you talked about human hearing and how humans perceive the direction of a sound. And of course that’s closely tied up with the shape of the head and the interaction of the sound against the person’s head as it enters the ear canal. What people call the head related transfer function, or HRTF. Are you relying then on the HRTF to get this done in your algorithm? And if you are, do you need any kind of calibration to determine the particular HRTF for any given person’s head? Size or can you work with a single generic model and that works for all people. So we are explicitly not modeling HRTF in our algorithm, but when we have analyzed what the algorithm is potentially leveraging, it is leveraging multiple different features in the signal itself. And that includes the way the head is actually reflecting signals. 1 of the intuition is basically that given that the separation between the microphones is pretty small, you want to still be able to localize each and every sound source. And the way to think about it is that your head, effectively, the reflections on your head, is effectively creating a virtual array which is larger than the separation between your ears itself. But the same time you don’t want to be in a situation where we go and fine tune the algorithm for each and every person’s head because that’s just going to be too much of an overhead. And in fact in our algorithms it was trained on, the training data was collected on like three to four people and then the testing was done on tens and tens of people who have never been seen the training data. No calibration of any kind. The algorithm automatically figures out, uses, figures out how to use the head related transfer function. And the way we ensure that the algorithm does it is that when we create a lot of synthetic data we get a robotic head which basically you can change the distance between the ears pretty significantly. And because we’re giving all that data, the algorithm is learning to figure that out automatically based on the sound itself without having us to calibrate for each and every person who’s wearing. The headset, which is a terrific feature of the algorithm that you can essentially deduce the HRTF or at least react to the individual HRTF and properly discriminate the distance of different speakers on the fly. And so if you have multiple speakers talking at the same time, two or three, you’re able to do that dynamically for all the speakers then? Correct, we can do that dynamically for all the, it’s plug and play. First, you don’t need to calibrate for any single person, person’s head. Second you can have multiple people. It’s basically letting all the people within the bubble through, including overlapping speech and everything else, which is extremely important by the way, because backchanneling is a very important way in which people communicate. When I say I agree and so on. These things which overlapping speech very, very important to convey intent and the person is on the same page as you or not on the same page as you. The third thing is that we also can deal with dynamics which people entering the bubble and leaving the bubble. So people can be outside the bubble, they can walk into the bubble and suddenly you can actually hear them. I mean there is like a delay of like maybe half a second to a second for it to basically start picking up. And people can leave the bubble and then you suddenly will not hear them. So it’s dealing with all these situations as well. So somebody enters the bubble while speaking. Your acquisition time is only half a second or so. That’s pretty impressive. Yep. And it’s, there’s nothing fundamental that it actually has to be half a second. It’s that we didn’t train the algorithms on mobility. So we just took the algorithms which were trained on static users and applied it to the mobility use case. And because of the specific ML components we use, like lstm, which have a huge amount of memory and because they have never seen any dynamic users in the training data the current algorithm has that delay, but you can always train the algorithm itself with mobility data to further reduce that particular response time. It’s a fairly edge case anyway that somebody’s going to be actively talking to you as they enter the bubble. But still even that half a second or so really is quite usable. Exactly. Now in a way you’ve kind of cheated because you did it with a headset. You’ve got six microphones in an array and you can get a lot done with six microphones in an array now and they’re connected with wires. So you don’t have any issue of latency or phase differences between the microphones. But now, and that would work in an office environment. You know, people wear headsets when they’re on Internet calls or even working when they want some quiet while they’re working in a, in a row of cubicles, for example. But you’re not going to get people to wear a headphone in a restaurant. They’re going to want to wear true wireless earbuds. So when you’re then limited to the microphones into two earbuds and you have to communicate wirelessly between them, will it still be possible to use this algorithm? Not this specific algorithm. So we submitted this paper almost a year ago. Its been going through reviews and since then we have actually been building wireless form factor devices which are low power and where you have microphones on just one side and microphones on this side, you’re communicating in real time. And there is also the multiple Issues. I think you kind of laid it out there. The first issue is that it has to be completely wireless. If you have a wired setup you have instantaneous access to all the microphones, microphone data which you can process. But if your microphones on the right ear and the microphones on the left ear, you really need to exchange information to get distance. But then there is an actual Bluetooth wireless latency which can be larger than the response time which you need to play back much quicker than the time it goes to send the data via Bluetooth from here to here. So that’s not going to be practical at all. So we had to come up with a different algorithm which we are going to publish in a few it’s not just going to be a single AI device. It’s going to be a couple of AI agents cooperating with each other with delayed information and can do. Yeah, there’s a lot of exciting things which are going to come out soon. So you’re going to basically see a wireless form factor device which is going to be power efficient as well. And it’s going to be in the form factor of a hearing aid. Lots of challenges to which we had to address over the last year to go from this headphone form factor device to something which is completely battery powered and a wireless hearing aid form factor. But wait for six more months. Its going to be all these things that you’re talking about are going to be in that form factor. Excellent And so the basic functionality be the same. Obviously the algorithm has got to be completely different for the wireless case of microphones in each ear, but the algorithm, the end result will be basically the same. Now I wasn’t going to bring up power consumption because the Raspberry PI is not meant to be power efficient. Right. It’s a desktop thing that’s a, that’s a hog on power. But the implication here is that you can run your model, or the new model for true wireless on something commercially available for earbuds like Sentient or Greenwaves is producing. Is that correct? I think that the implication of the work we put out there already in public is that you don’t really need huge GPUs. Raspberry PI has very limited computation. The model size we have is less than half a million, half a million parameters which is pretty small compared to the kind of think about ChatGPT kind of models. They are like. You can have a lot of parameters when you have a cloud behind you. Right, but half a million parameters, right, they’re capable of swallowing a half a million parameters. But there is also the other issue that when just counting the number of parameters is not good enough is what we have learned when we work with some of the state of the art AI chips or headphone chips for example, because you can have really, really complex operations like LSTMs and so on in ML which have a least number of parameters but are computationally not supported because they are very complicated. So you have to also redesign the whole neural network itself so that it can run on these custom because these custom low power accelerators, AI accelerators people are designing for headphones and stuff have been designed a few years ago and by the time the next generation of these things come out, it’s going to be at least three to four years because hardware moves much slower than software itself. But we have now finally, one of the things which I was going to mention it’s going to come out is that that’s another big challenge we have had to solve which is going to come out soon. Okay. Okay. This can be really exciting in it. It’s just not like, oh, I can take this algorithm and run it on these platforms. Primarily because when you went out starting off it’s like, oh, we only have half a million parameters. Of course we can run it on your, on, on these kind of platforms. But then it turns out that, oh, that does, that number doesn’t. I mean you don’t want to get a billion parameters. You can’t do that anyway. But just because you’re less than a million parameters doesn’t mean that you can run it on these platforms because you could be using operations which are not amicable to these low power AI accelerators. So you need to rethink the architecture. So we had to come up with, yeah, we are coming up with a completely different architecture to solve these problems. But yeah, if you ask me in January if you would have been able to gotten this on a wireless earbud or like a wireless hearing aid, I would have been like maybe in five years. I can tell you that we are a month away. Okay. No, that’s really, that’s exciting stuff. And yes, it’s clearly symbiotic. As a general guideline your models are small enough to pull this off, but yeah, you have to design completely for the chip. I think most people listening here will understand that and wait with bated breath to see how this works in a TWS. Because when you think about it, there are actually quite a few people who don’t have hearing loss but have trouble hearing in noise. Theres a couple of studies. Dr Beck and Dr. Edwards have both said that in the United States alone, there’s about 25 million people who complain of hearing difficulty but don’t have audiometric hearing loss. So even in a TWS implementation that gives 10 or 15 dB of SNR improvement. This is huge for that person. Absolutely huge. So it’ll be exciting to see how your implementation in a TWS actually turns out. Now you’re actually providing the source code and the training data sets in the public domain. But then in the Washington University article it mentioned that you’re going to start up a company to take this to market. So what does the future actually look like given that you’re going to have open source algorithms and open source training data and at the same time you’re going to start a company to take this to market. So what does the future actually look like given those two things? So we made the code open source so that other researchers. It’s not for, it’s only for non commercial, it only has a non commercial license so that we can still make sure that researchers actually can build on it. The algorithms itself. One of the challenges with this hearable space is that it really requires experience in both hardware and software. And when I say just say hardware. It just doesn’t mean that I’m going to create a pcb. But you need to understand AI algorithm accelerators and stuff. Its not that easy it’s not that easy for everyone to basically just be like, oh, I have the code, I can basically replicate it. Thats basically the reason why if I was in a space where anyone could just replicate it, I would have been like, just put it out there open source and not really think about commercializing it. Because if anyone can do it, then you don’t need to spend time trying to commercialize something which anyone can do. So yeah, the code is basically put out there for non commercial on a non commercial license. And the data set, the synthetic data sets and stuff is all non commercial. But if you want access to the real world data, it turned out that there is no distance based real world data. So we had to build a whole robotic platform to collect it at a large scale. That is not public. You have to Reach out to us and we only give access to you if it’s a non commercial research only use case. But I think taking a step back, the bigger issue here is that as you know in the hearing space one of the reasons why AR hasn’t really taken off is because you’re constrained by the compute and the real time requirements of something like this. You need to process your audio within less than 8 milliseconds. In contrast to ChatGPT where you can just give it a query, hey, who’s the president of blah? And it’ll take a few seconds to respond, it’s fine. But here you need to keep processing continuously and playing it back within less than 10 milliseconds, maybe 4 milliseconds. And if you really want it to be hearing aid compatible and that makes it really challenging for people to get into it. And the second bigger issue is also that the hardware constraints, you need to understand what hardwares can actually do. And once you go from phones, what phones can do, to what Orange PIs can do, to what Raspberry PI can do and finally to what AI accelerators at this tiny scale can do, it’s a lot of learning curve. And as a result like I think there is a big need for people who have expertise across all these things like creating these kind of cocktail party problem solving things as well as understanding the hardware constraints, building the actual complete hardware prototyping, understanding the user requirements, having expertise in creating the actual algorithms, hardware, the whole stack the number of people who have that thing is pretty limited. So if you want change to happen quickly in the space, one of the only easiest ways to do that is do it yourself, right? No, that makes perfect sense. So what it comes right down to it is that the code you used in the headphone and the commercially available data sets, training data sets aren’t going to get you very far in their own. And buried in there was something which sounds pretty significant and that is there were no commercially available distance related training sets so you actually had to create your own. And so that’s some of your key IP you’re not going to let out except under controlled circumstances. That as well as the fact that we have all the neural networks which we are designing for hearing aid and wireless earbud form factor devices which are different from what is published, which are going to be, which we didn’t realize that it’s going to be different and now it’s going to be to share and like it’s a completely different architecture now to get it to a wireless thing which is going to be power efficient. So there is not just data set but also there’s a whole figuring out how to do quantization. There’s a whole thing here which is because you can’t really run things on floating point. I mean I don’t want to, want to go into technical details here but like there’s so much technical details of getting it into a power efficient and a wireless form factor device where the existing things we made public don’t really, you can’t just take them and make it work. It’s years and years of work to get there. So if you want to accelerate that by five years for example, we can make that happen. So I think that’s basically what we see as an opportunity for accelerating the adoption of this technology. We are developing into every airport, every hearing aid out there so that people can use it. Because I want to use it. All my friends, they’re like, I want to use this as well. So I’m like, I want to be part of the company or like be something which actually I’m creating and be like oh, I created the bubble. You know, I’m saying you’re using the bubble and I created that. Yeah, I understand the total stack is really much more complicated than what you’re sharing on open source and you’ve really accomplished a lot. Very exciting stuff you want to add. Any last thoughts before we wrap it up? I do think that taking a step back people think of hearables and hearing aid as just improving hearing for example or enhancing hearing for people who have hearing loss or difficulty in hearing. But I also think that hearables are a very natural interface between AI and humans. Primarily because culturally it’s so acceptable for us to walk around wearing headsets and AirPods and like hearing aids and everything. You can just walk around on the street with airports, you’re not going to get dirty looks. But if you take a camera with the glasses and then walk around on the street, people are going to give you that or walk to a bar, people are going to be like are you recording me right now? Like it’s – it’s, it’s not the same social acceptance people have with cameras in your head, on your, on your head or like a glass form factor device which has cameras. So I do think that hearables beyond hearing, hearables are the natural interface between Humans and AI and thinking about what that interface creates and how that can augment humans senses, hearing capabilities of intelligence and creativity and memory. All. There’s lots of things you can augment using this particular interface which I think is an exciting space to be in for hearables in general. Because this is a very natural and acceptable interface which people already wear. You don’t need to create new habits for people. People already have the habit of wearing headphones and like AirPods. Now the question is how do you create exponential capabilities used based on this habit which already people have. You don’t need to train them to get glasses and train them to wear like Apple Vision Pro, for example. So I do think that the kind of things people can do with hearables go far beyond just enhancing hearing. And that’s where I think this is a very exciting space to be in, I think. Well you know, when this podcast is published, there’s going to be a person named Dave Kemp waving his arms back and forth like this because he and I have had this conversation about the value of hearables, especially using a voice interface as the interface for years now. And it’s perfectly true that using a visual interface for conversational AI is much more difficult than using a voice interface. Once the large language models get better in, voice interface becomes more natural, more accurate, more responsive and also more context aware. It’s going to be the interface. And so all of the different features that are coming together, health related features AI and language model related features and hearing related features are all converging and that’s the exciting future. So congratulations on being a part of it and bringing value to that through what you’ve developed here. So now really appreciate it and thanks a lot for joining me on the podcast and thanks everyone for listening or watching to this episode of this week in Hearing. Thanks a lot. Thanks a lot, Andrew.
Resources:
Be sure to subscribe to the TWIH YouTube channel for the latest episodes each week, and follow This Week in Hearing on LinkedIn and on X (formerly Twitter).
Prefer to listen on the go? Tune into the TWIH Podcast on your favorite podcast streaming service, including Apple, Spotify, Google and more.
About the Panel
Shyam Gollakota, PhD, is a professor of computer science and engineering at the University of Washington, specializing in wireless systems, digital health, and artificial intelligence. His pioneering work includes the development of battery-free devices, health monitoring systems, and AI-driven “sound bubble” technology to enhance communication in noisy environments. Recognized globally, he has received accolades such as the ACM Grace Murray Hopper Award and has been named one of MIT Technology Review’s 35 Innovators Under 35. Dr. Gollakota’s research bridges cutting-edge innovation with practical applications, driving advancements in healthcare and wearable technology.
Andrew Bellavia is the Founder of AuraFuturity. He has experience in international sales, marketing, product management, and general management. Audio has been both of abiding interest and a market he served professionally in these roles. Andrew has been deeply embedded in the hearables space since the beginning and is recognized as a thought leader in the convergence of hearables and hearing health. He has been a strong advocate for hearing care innovation and accessibility, work made more personal when he faced his own hearing loss and sought treatment All these skills and experiences are brought to bear at AuraFuturity, providing go-to-market, branding, and content services to the dynamic and growing hearables and hearing health spaces.